Every so often, I come across studies that leave me scratching my head. Sometimes, these studies are legitimate scientific studies that have huge flaws or come from an assumption that is very off-base. Other times, they involve what Harriet Hall has termed “tooth fairy science,” wherein the tools of science are used to study a phenomenon that is fantastical, whose very existence hasn’t been demonstrated. Many such studies, not surprisingly, are studies of “complementary and alternative medicine” (CAM) or “integrative medicine” (IM). Modalities like reiki (which is faith healing that substitutes Eastern mysticism for Christian beliefs) and homeopathy (which is, when you boil it down to its essence, sympathetic magic) fall into the category of therapeutic modalities that are based on fantasy but are studied as with the latest tools of science, producing no end to confusing noise. This “tooth fairy science” has, over the last few years, reached its epitome in the application of the latest genomics technology to, in essence, magic, and I’ve recently come across an incredible example of just such a thing. But, first, let’s take a step back to what is going on in medical science now before I introduce a concept that I’ve dubbed “woo-omics.”
A prelude to woo-omics: Genomics, proteomics, everywhere an “omics”
One of the most difficult problems in science-based medicine is how to do a better job identifying which patients will respond to which treatments. Clinical trials, by their very design, have to look at average responses in populations. In essence, a treatment is compared to either placebo or standard-of-care, a choice mainly driven by ethics and whether effective treatments exist for the condition being studied. It is then determined using statistics whether a significant difference exists between the two groups. The difficulty, as any clinician knows, is applying the results of clinical trials to individual patients. In any population, there is, after all, a range of responses to any drug or treatment, and it would be desirable to be able to predict which patients will fall at the end of the bell-shaped curve where the treatment is most effective and which will fall at the end of the curve where the treatment works poorly or not at all.
In the past, predictors of response (or, just as importantly, lack of response) to any given therapy were crude. For instance, take my specialty of breast cancer. If the tumor does or does not express the estrogen receptor (ER) determines whether it will be sensitive to antiestrogen therapy. It also can to some extent predict whether a tumor will be responsive to chemotherapy, as ER(+) tumors tend to be less responsive to chemotherapy than ER(-) cancers. However, that’s not entirely true in that there are subsets of ER(+) tumors that are responsive to chemotherapy. It’s just that, until recently, we couldn’t identify them other than by administering adjuvant chemotherapy and observing the response. Fortunately, in the last several years, clinicians have started to get some help in this area. For example, a 21-gene expression assay known as the Oncotype DX assay can determine whether an ER(+) tumor is likely to be responsive to chemotherapy or not. Basically, the test looks at a panel of genes and produces what is known as a “recurrence score.” Low recurrence scores mean a lower likelihood of recurrence and less benefit from chemotherapy, while high recurrence scores indicate a higher probability of recurrence and a greater benefit from chemotherapy. As a result, the primary tumors from most women with ER(+) cancer that has not as yet metastasized to the axillary lymph nodes are tested with Oncotype DX, and patients whose tumors have low scores are not offered chemotherapy, thus thwarting what Mike Adams calls the “breast cancer industry” and its relentless search for profits by pumping women with breast cancer with expensive and toxic drugs. Oh, wait, it was scientists and doctors who came up with the Oncotype DX test. Damn, we’re good at working against our own interests and those of our pharma masters.
Be that as it may, these days, the search for predictors of response, prognosis, and therapies most likely to do good has moved into the realm of what we now call “omics.” The term “omics” as it is used today originally came from genomics, which is, put very simply, the study of the entire genome (i.e., all the genes in an organism). It then expanded to be used for proteomics, which, again put very simply, is the study of all the proteins expressed by a cell type, organ, or organism. Since then, the term has metastasized to many, many areas of biology, such as metabolomics, secretomics, lipidomics, and many, many others. Here’s a general schema of what I’m talking about:
The problem with all these “omics” is that they are hideously complicated, with interactions of thousands of genes, proteins, and other entities that must be made sense of in order to understand what is going on. Indeed, arguably the reason we never bothered with these sorts of analyses before is that, until the last 10-20 years quite simply they were impossible. The computing power and algorithms necessary to do them simply didn’t exist and had to be developed. Neither did the technology. Then, beginning in the late 1990s, techniques were developed to measure expression profiles that included every known gene in the human genome. Building on techniques developed for the Human Genome Project and other genomics initiatives, in the early 2000s, we had cDNA microarrays, the ability to scan thousands of single nucleotide polymorphisms (SNPs) and look for associations with diseases, and the like. Systems biology started to come into its own, wherein biology was studied not so much at the level of the single gene and protein but by looking at expression and activity data and constructing networks that look like this:
The result of the new systems biology and “omics” has been a torrential flood of data that’s far ahead of our ability to analyze it fully. As the cost of sequencing a genome has fallen from hundreds of thousands of dollars to less than $10,000 (soon to be less than $1,000), genome sequencing will soon fall to within the price range of other commonly used medical tests. (CT scans and MRIs cost around $2,000 or so, and the Oncotype DX test, for example, costs around $3,000.)
Unfortunately, even as the flood of data accelerates, successful strategies for actually using that data clinically have been elusive. Indeed, last year, around the time of the tenth anniversary of the completion of the Human Genome Project, there were a series of articles asking, basically, “Where are all the cures we were promised?” Of course, as I’ve pointed out before, the sequencing of the human genome (and now all these other genomes, as is being done in the Cancer Genome Atlas, for example) has been the easy part. The hard part is making sense of it all and relating differences in individual genomes to specific diseases and to the discovery and validation of biomarkers for response to specific therapies. Just looking at one example can demonstrate why it’s so hard to make sense of this data and to figure out how to use it to develop cures to diseases like prostate cancer. Does all of this mean that all the information we’ve gathered and connections we’ve made so far in the Human Genome Project, the Cancer Genome Atlas, and other similar projects that have tried to relate genomics data to human disease, prognosis of disease, and response to therapies useless? Of course not. It’s just that the speed with which this data will result in real cures was arguably oversold. Right now, the situation is confused and uncertain. and we are still very far from the vision of truly personalized medicine that so many see “omics” as the path towards.
Which makes it perfect for purveyors of unscientific medicine to leap in and take advantage of. First, I’ll introduce the concept of “woo-omics” (which, to my knowledge, is a term coined by me to refer to the application of omics principles to woo). Then I’ll show you a truly ridiculous example, after which I’ll try to bring it all together at the end.
Woo-omics
Promoters of “complementary and alternative medicine” (CAM) and “integrative medicine” (IM) realize that, if CAM/IM is ever to be taken truly seriously they need to provide a patina of scientific respectability to it. Whether it deserves that respectability or not is secondary to the endeavor of promoting unscientific medicine as being co-equal with science-based medicine (SBM). Also, promoters of CAM/IM are nothing if not mimics. They’re very good at jumping on the latest scientific bandwagon as a means of trying to achieve this patina of scientific respectability. Not surprisingly, believers in CAM/IM, aided and abetted by the National Center for Complementary and Alternative Medicine (NCCAM) have jumped on the “omics” bandwagon in general and the genomics bandwagon in particular. I first became aware of this four years ago, when I noticed an RFA from NCCAM for omics studies in woo entitled Omics and Variable Responses to CAM: Secondary Analysis of CAM Clinical Trials:
This initiative will be used to stimulate omics analysis by CAM investigators. Multiple NCCAM and other NIH CAM clinical studies have appropriately banked patient samples from several sources including a variety of tissues. Therefore, this initiative will encourage use of these already acquired samples from well-designed clinical studies for identification of genomic, proteomic and metabolomic and other omic variants that may be markers or classifiers for the level of response to CAM interventions. Trials need not exhibit significant differences between arms. This analysis may be applied to studies with negative results as a function of high patient variability in the treatment arm, which may represent responder/nonresponder phenotypes. Since this work would use samples already collected, the participants may not be consented for genetic studies and would have to be reconsented and samples de-identified. Guidelines for data access and release can be found at http://www.fnih.org/GAIN/GAIN_home.shtml.
“Well-designed clinical studies”? You keep using that term. I do not think it means what you think it means. Of course, any project proposed for this RFA would by its very nature have to be a massive fishing expedition. As much as I like the new technology that can produce such copious amounts of data, I’m not a big fan of such studies even in conventional medical trials, where the treatment studied has clear efficacy, a molecular mechanism of action, and thus a reason to think that there might be “omics” profiles that correlated with magnitude of response. For one thing, such studies are not hypothesis-driven, and investigators will not have much of an idea of what they might expect to find; that is, if they find anything at all. Thus, in the absence of clear criteria for what constitutes a significant finding, there is a significant risk of all sorts of spurious findings being labeled as “significant.” Of course, if researchers look for a large enough number of correlations, they will inevitably find them even if they are not true indicators of causation just on the basis of random chance alone. Such analyses are in essence post hoc exercises; it is far better to build such analyses into a study prospectively from the very beginning. The data is cleaner that way, and the statistical analysis has more power.
That being said, I will concede that for some clinical trials, post hoc analysis of the tissue specimens can be very useful as a means of generating hypotheses. Specifically, if there are clear-cut “responders” and “nonresponders,” subjecting samples from these patients to “omics” analyses can provide clues to help investigators determine why some subjects respond and some do not. Useful hypotheses to test can be generated from such correlations. In fact, this is the very reason why I found the NCCAM proposal so pointless: precious few of the modalities tested in NCCAM-funded grants have ever been shown to have even a glimmer of efficacy greater than that of a placebo, and when you start talking about reiki or other “energy healing” techniques, homeopathy, or various other techniques with no clear basis in science or reality, you’re talking about magic. Given that, it’s a waste of resources, specifically precious grant money that could go to fund far more worthy endeavors. While this sort of approach might be somewhat useful for various herbal remedies, mainly because such remedies are drugs (impure drugs with many components, but drugs nonetheless), they would be utterly useless for practically every other CAM modality. For example, does anyone imagine that genomics, proteomics, or any other “omics” would identify “responders” and “nonresponders” when it comes to Reiki therapy. I don’t think so–unless there is an “omics” profile for credulity or the placebo effect. Or maybe they will discover a genomic or proteomic profile for credulousness or personal belief structure.
Fortunately, with the hindsight of four years, I’ve only been able to find two projects funded under this mechanism. Unfortunately, they are grants entitled Omics and variable responses to placebo and acupuncture in irritable bowel syndrome and Dietary calcium and magnesium, genetics, and colorectal adenoma. The former project is based at Harvard, specifically at Beth Israel Deaconness Hospital, and refers to trying to determine biomarkers of response to acupuncture therapy in patients with irritable bowel syndrome using -omics technology applied to blood samples:
Irritable bowel syndrome (IBS) is a chronic medical condition which affects 10-15% of the population in industrialized countries. IBS is associated with a profound impairment of patient’s quality of life, significant loss of productivity, and $2 billion in direct health care costs in the US alone, mainly due to unsatisfactory treatment. Patient heterogeneity in terms of disease pathogenesis, symptom expression and psychological co-morbidities are among the factors implicated in the variation of therapeutic responses. Therefore, the need to develop biological markers that can distinguish patients likely to benefit most from specific forms of treatment, and as importantly, from a placebo treatment, is imperative. We have recently concluded a 6 week randomized clinical trial in IBS patients (n=262) to examine the effects of placebo and acupuncture treatments, compared to a natural history control group. Blood samples were collected at baseline and at 3 and 6 weeks of follow-up. The positive results of this trial and sample availability allow us to perform a secondary Omics analysis examining patient variability in response to treatment. Our primary hypothesis is that serum proteins participate directly or indirectly to the brain-gut interactions associated with clinical responses in IBS. Our second hypothesis is that patients who clinically respond to placebo or acupuncture treatment have distinct serum protein profiles from those who don’t respond. A third hypothesis is that placebo or acupuncture treatment results in changes in the serum proteome. We will incorporate various high throughput experimental approaches, some of them targeted to specific biological pathways and others leading to unbiased discoveries, to pursue the goals of this study. The serum proteomics analysis will be complemented with an investigation of common polymorphisms in genes of interest that emerge. Findings stemmed from such studies could lead to improvement of inclusion/exclusion criteria in randomized clinical trials based on biological screens. In terms of personalized medicine, findings could result in methodologies to enhance medication responses, adjust the doses or select medications with fewer side effects.
The study used as the basis in preliminary data for the above grant appears to be Components of placebo effect: randomised controlled trial in patients with irritable bowel syndrome. Guess who the first author was before clicking on the link. Go on, guess. OK, I’ll tell you: Ted Kaptchuk. And what was the finding of the study? Basically, it looked at IBS patients treated either with sham acupuncture or acupuncture plus “augmented interaction” with the practitioner. In the former group, acupuncturists spoke little with the patients and interacted with them as little as possible, while in the latter group they spent a lot of time with the patients asking them about their lifestyles and various other issues. Basically, both of these groups did better than the observation-only control group, while the “augmented interaction” group demonstrated more improvement in symptoms. It was actually not a bad study, although all it showed was that sham acupuncture works as a placebo and that a closer interaction with health care practitioners enhances the placebo response, which is nothing new.
As for all the specimens, given that the placebo acupuncture group and the “augmented interaction” groups were re-randomized half way through the trial, it’s hard to see how applying omics technology to these samples is likely to provide much useful information. Moreover, the design of the trial incorporated a nested substudy of true acupuncture that allowed the investigators to tell subjects truthfully that, if they were randomized to an acupuncture group, they had a 50% chance of receiving “true” acupuncture. The results of this sub-study were ultimately reported in a psychiatry journal and in a followup article in the American Journal of Gastroenterology. Interestingly, the latter report says in essence nothing about whether true acupuncture produced better results than sham acupuncture. Indeed, Kaptchuk goes out of his way to avoid discussing that question in the article and even lumped together acupuncture and sham acupuncture groups when assessing “responders” and “non-responders,” which strongly suggests to me that there was no difference between the sham and “verum” acupuncture groups.
And Dr. Efi Kokkotou, along with Ted Kaptchuk, propose to do omics studies on blood specimens from these subjects, even though there was no real effect due to the intervention and even though the intervention group was re-randomized at three weeks. I’m not sure what Kokkotou and Kaptchuk think they’re going to find, but with multiple comparisons I’m sure they’ll find something and that that something is very likely to be noise or spurious results.
All to the tune of $521,646 in FY2011 and a total of $1,514,997 thus far, your tax dollars hard at work again, courtesy of NCCAM. Interestingly, listed as one of the publications resulting from this particular grant is a study I discussed earlier this year in which Kaptchuk and company tried to claim that they could harness placebo effects without deception. As much as they tried to spin it otherwise, they couldn’t. Another paper whose work was funded through this grant claims to have found a serum correlate of placebo response in IBS. One sentence completely undermines this claim:
Since the current study was hypothesis generating, we did not apply Bonferonni/Dunn correction for multiple comparisons to any of our analyses.
Sorry, but being “hypothesis-generating” is not an adequate reason not to correct for multiple comparisons. One wonders if Kokkotou and Kaptchuk will apply the same logic to omics data. If they do, they’ll have at least hundreds of potential “biomarkers.”
While the abuse of omics technology by researchers like Kaptchuk (and, by the way, Dean Ornish as well) is egregious, I had no idea that I hadn’t seen the worst of it.
And now…Ayurvedomics!
I don’t remember how this came to my attention, but it did. It’s an article that appeared in ACS Chemical Biology entitled Ayurgenomics: A New Way of Threading Molecular Variability for Stratified Medicine. I kid you not. It’s courtesy of Mitali Mukerji at the Institute of Genomics & Integrative Biology (IGIB) in New Delhi, India. Her webpage on the IGIB website even lists her interests as the application of polymorphisms in mapping mutations and disease origins in SCAs; Ayur-genomics: exploring the principles of predictive medicine in Ayurveda; and Alu elements in genome organization and function. It all sounds so science-y, and—who knows?—interest numbers one and three sound reasonable enough. But interest number two? Not so much. Get a load of the rationale for the article. After discussing the difficulties in genome-wide association studies in “pinning down human physiology to a few loci,” Mukerji writes:
We found this challenge very stimulating, and our understanding of population-wide variability across Indian populations (IGV Consortium)(6) provided a major thrust to further our quest for understanding the variability in healthy individuals. The head start came from the fact that there exists an exquisitely elaborate system of predictive and personalized medicine in India, i.e., Ayurveda, which has been practiced for over 3500 years. The system already has a built-in framework for stratifying healthy individuals who differ in susceptibility to disease and response to drug and environment. In contrast to the empirical approach of contemporary medicine, the Ayurveda therapeutic regimen is tailored to an individual’s physiology. Though this system has fueled many drug discovery approaches and some attempts into its integration in pharmacogenetics have been made,(7, 8 ) a systematic analysis of underlying principles has been lacking. In order to undertake integration of this most ancient system of medicine, which is scripted in Sanskrit, with the language of modern genomics and medicine, we undertook this endeavor with a trans-disciplinary team of researchers. For the first time we could demonstrate molecular evidence for these concepts and build a framework for “Ayurgenomics”, which can provide impetus to personalized medicine.(9, 10) We provide a perspective of the concepts and also the further prospects in this field (Figure 1). The original Sanskrit verses with their meanings are available as supplementary online material in our prior publications.
Now, it was news to me that Ayurveda had “fueled many drug discovery approaches”; so I looked up the two articles referenced. Like Mukerji’s article, these references looked at the three major constitutions postulated in Ayurveda under the Prakriti classification. One article was in a woo journal of highly dubious value and postulated a “reasonable” correlation between Prakriti classification and certain Prakriti types, while the other article was a commentary suggesting using Prakriti and asserts that the “concept of Prakriti or human constitution plays a central role in understanding health and disease in Ayurveda, which is similar to modern pharmacogenomics.” Basically, the whole article argues that, because a few useful compounds from Ayurveda, that Ayurveda could be useful as a basis for drug discovery and the development of “personalized medicine.”
Not knowing what the heck Prakriti is, I decided to look it up. Thanks to Wikipedia and other sources, I now know that in Hinduism Prakriti is the “basic nature of intelligence by which the universe exists and functions” and the “primal motive force.” In Ayurveda, it’s used to refer to a body type in Ayurveda. Here is a test to determine one’s Prakriti. Based on the results, one is classified according to three Doshas: Vata, Pitta and Kapha. These three Doshas can be combined into ten ways to produce ten body types. Now, certainly some of these characteristics likely have something to do with health (“overweight, difficult to lose weight,” for instance, or “fair skin, sun burns easily,” the latter of which could indicate susceptibility to skin cancer). However, just because body types based on a system of classification that is more or less based on an Indian form of vitalism (“primal motive force” or “basic nature of instelligence”) might coincidentally have something to do with discoveries found later does not mean that the basis of the system upon which these body types are based has any external scientific validity. One might as well perform omics analyses on people to predict responses to homeopathy.
Yet none of this prevents Mukerji from proposing this system for “weaving the threads of molecular variability through Ayurgenomics”:
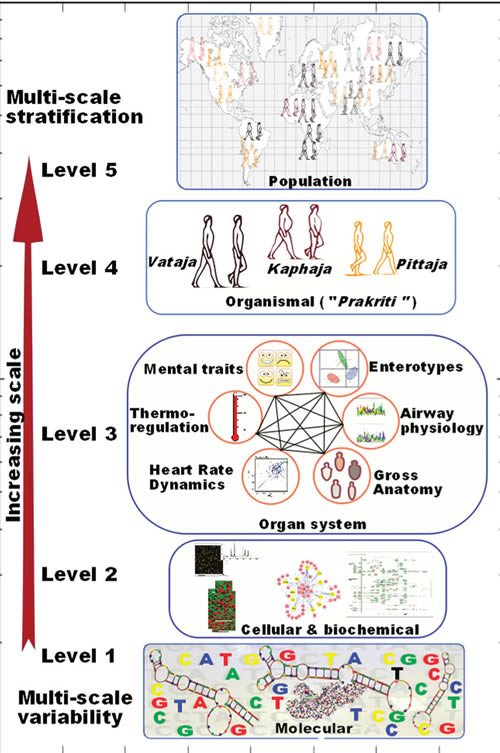
This is proposed even though Mukerji basically points out that Prakriti is basically very much like the four humors in “Western” medicine and the five elements in traditional Chinese medicine:
Ayurvedic practitioners deconvolute the “mixture impression” thus obtained to identify proportions of Vata, Pitta, and Kapha in an individual’s Prakriti. This “subjective” assessment (which was objectivized to a scoring system through a questionnaire) considers different phenotypic attributes of an individual and links these multiple windows to create intraindividual phenotype-to-phenotype links. A disease according to Ayurveda is a perturbation of Vata, Pitta, and Kapha in an individual from his or her homeostatic state. Ayurvedic treatment aims to bring it back to its native state by appropriate dietary and therapeutic regime.
And:
Ayurveda describes not only the functional attributes of Vata, Pitta, and Kapha but also their contribution on different scales in seven different constitutions. Therefore, Ayurveda already has a stratified approach as its basic tenet for personalizing therapy. Thus we felt that integration of this stratified approach could complement approaches to development of personalized medicine while gaining insights into systems biology. We realized that before making any attempt toward this endeavor we would have to address an ontological challenge in connecting together the literature from Ayurveda, modern medicine, and molecular biology.
Again, combining Ayurvedic literature with modern medicine and molecular biology would be a good thing is not explained. To me, that would be a lot like taking the writings of Hippocrates and combining them with the latest cutting edge molecular and systems biology techniques. It would be highly unlikely to be informative or helpful.
Not surprisingly, Mukerji cites a lot of her own work in this review article, including an article from 2008 entitled Whole genome expression and biochemical correlates of extreme constitutional types defined in Ayurveda, which Steve Salzberg, a systems biologist and skeptic, looked at in 2008. He was not impressed, and neither was I when I read the paper. Basically, Mukerji and her co-investigators partitioned subjects into the three Doshas based on Ayurvedic principles, but in reality the vast majority of the classification is simply based on body type and body habitus. That’s why, when the authors found that genes associated with an elevated risk of cardiovascular disease are more highly expressed in Kapha males, it’s not particularly surprising, given that Deepak Chopra and others characterizes Kapha as prone to obesity. In other words, so what if Mukerji found differences in gene expression based on Ayurvedic body types. They’re different body types, and we already know that people with different body habitus can be prone to different kinds of diseases. Ayurveda adds nothing new to this knowledge, much less suggests a rationale upon which to base a new “omics” discipline. Taking studies like this and proposing something as ridiculous as “Ayurgenomics” does nothing more than show how far believers in pseudoscience will go to try to conjure up a seemingly scientific justification for their woo. Remember, Doshas resemble, more than anything else, the Indian version of the Four Humors. Do we try to fit humoral theory into a new “omics” discipline? No, we don’t, although I fear that some day someone might try.
Unfortunately, as Steve Salzberg points out, NCCAM is funding grants like R21AT001969, which funds the “Ayurvedic Center for Collaborative Research.” A quick search for NCCAM grants funding Ayurveda on NIH Reporter reveals several grants in Ayurvedic medicine funded by NCCAM, including Ayurvedic alternatives in autoimmunity and A whole systems approach to the study of Ayurveda for cancer survivorship. One wonders how long it will be before NCCAM starts funding “Ayurgenomics” projects.
Woo-omics ascendant?
The whole idea of doing omics on tissue samples from NCCAM-funded studies is about as good an example of putting the cart before the horse as I can think of. There is no doubt that individual biological variability is problematic for all medicine and is the main reason that clinical trials of sufficient size and power are needed to separate random biological variation from real treatment effects. However, if a treatment is efficacious in a significant part of the population, it should demonstrate efficacy in a properly designed clinical trial, and if there are significant differences in how people respond sufficient to produce two classes (responders and nonresponders) that should show up as well. Studying omics in the absence of clear evidence that these two conditions apply (clinical efficacy and definite groupings of responders) is about as likely to produce useful information as a 30C homeopathic remedy is to contain a single molecule of the original remedy upon which it’s based. Similarly, trying to yoke genomics to a system of religion-inspired prescientific “medicine” like Ayurveda is no different than proposing to base genomics profiles on the four humors.
The really frustrating aspect of woo-omics is that, in these constrained budgetary times, any money devoted to such studies is money diverted from more scientifically worthy projects. A justification can be made for such retrospective analyses when there is definite evidence of efficacy and definite evidence of classes of responders and nonresponders, as is found in many drug trials, but, barring that, it’s far more likely that good money will be thrown down the pit after bad to find out whether there is an “omic” profile for woo. Worse, it’s already difficult enough to validate candidate biomarkers based on existing omics data. Between measuring the expression of every messenger RNA and microRNA in a cell simultaneously and performing next generation sequencing and various other techniques that permit the sequencing of whole genomes and expression data simultaneously, we can measure more things in a cell than ever before. However, because we can measure so much, it’s all just noise when there is no actual effect detected between two treatments, and it’s a lot of potential noise because thousands of comparisons are being made simultaneously in even a simple comparison. That’s why adding magic to the omic mix will not help, as it will only accentuate the noise. Unfortunately, magic is exactly what defines woo-omics.